Table Of Content

However, building, maintaining and searching fully enumerated chemical libraries comprising more than a few billion compounds become slow and impractical. Such gigascale virtual libraries are therefore usually maintained as non-enumerated chemical spaces, defined by a specific set of building blocks and reactions (or transforms), as comprehensively reviewed in ref. 38. Within pharma, one of the first published examples includes PGVL by Pfizer37,43, the most recent version of which uses a set of 1,244 reactions and in-house reagents to account for 1014 compounds. Other biopharma companies have their own virtual chemical spaces38,44, although their details are often not in the public domain. Among commercially available chemical spaces, GalaXi Space by WuXi (approximately 8 billion compounds), CHEMriya by Otava (11.8 billion compounds) and Enamine REAL Space (36 billion compounds)45 are among the largest and most established.
Molecular Modeling of Proteins: Methods, Recent Advances, and Future Prospects
A binding-site identification method under the SILCS framework, named SILCS-Hotspots, was developed recently by our laboratory (115). SILCS-Hotspots is designed to identify fragment binding hotspots that are spatially distributed across the global protein structure including both surface and interior binding sites. The general protocol using SILCS-Hotspots to identify putative binding sites on a protein is described as the following. With the fast development of more powerful computing hardware, expensive algorithms such as free energy perturbation methods (23), which can only be used to finely tune the drug candidates at the lead optimization stage, become much more affordable and have been routinely used in a range of applications (24–26). Alternative CADD methods represent novel solutions that exploit the interactions between drugs and targets are also seeing wider use. Our laboratory put forward the SILCS methodology as described previously, and information from SILCS can be utilized in many different ways in various aspects of drug discovery (16–18).
Challenges of gigascale screening
To avoid this resynthesis, it has been suggested to use ML modes trained on DEL results for each target to predict drug-like ligands from on-demand chemical spaces, as described in ref. 36. Moreover, it presents structure- and ligand-based drug design tools to optimize known drugs and guide the design of new molecules. The book also describes methods for identifying small-molecule binding pockets in proteins, and summarizes the databases used to explore the essential properties of drugs, drug-like small molecules and their targets. In addition, the book highlights various tools to predict the absorption, distribution, metabolism, excretion (ADME) and toxicity (T) of potential drug candidates. Any computational tool based on pre-defined algorithms and scripts has its limitations, and the computational tools/methods used in CADD, such as, molecular docking, virtual screening, QSAR, pharmacophore modeling, and molecular dynamics, have their own limitations [49, 85-88]. Furthermore, ADME and many toxicity prediction tools are not supported by solid experimental data, and many examples of the failure of these computational approaches can be found in the literature [89, 90].
Senior Principal Scientist, Computational Medicinal Chemistry
This mishap exemplifies the need for a better understanding of behaviour of DL models and their dependence on the training data, which is widely recognized in the AI community. It has been shown that DL models, especially based on limited datasets lacking negative data, are prone to overtraining and spurious performance, sometimes leading to whole classes of models deemed ‘useless’88 or severely biased by subjective factors defining the training dataset89. Statistical tools are being developed to define the applicability range and carefully validate the performance of the models. One of the proposed concepts is the predictability, computability and stability framework for ‘veridical data science’90. Adequate selection of quality data has been specifically identified by leaders of the AI community as the major requirement for closing the ‘production gap’, or the inability of ML models to succeed when they are deployed in the real world, thus calling for a data-centric approach to AI91,92. There have also been attempts to develop tools to make AI ‘explainable’, that is, able to formulate some general trends in the data, specifically in the drug discovery applications93.
The pharmacophore model generated should have optimum sensitivity and specificity to minimize the chances of false negative and false positive results and must be validated using an independent external test set [81]. If the information about the 3D structure of a receptor and a set of known active compounds are lacking, then a sequence-derived 3D pharmacophore model is quite useful. For example, Pharma3D utilizes knowledge of the 3D crystal structures and homology models to derive the common sequence motif important for receptor-ligand biomolecular interactions in protein families [81, 82]. Running such data-rich computationally driven pipelines requires overarching data management tools for drug discovery, many of them being implemented in pharma and academic DDD centres146,147.
Meet the UC Campus Leads
Especially impressive has been the recent structural turnaround for G protein-coupled receptors (GPCRs)17 and other membrane proteins that mediate the action of more than 50% of drugs18, providing 3D templates for ligand screening and lead optimization. The second factor is a rapid and marked expansion of drug-like chemical space, easily accessible for hit and lead discovery. Just a few years ago, this space was limited to several million on-shelf compounds from vendors and in-house screening libraries in pharma. Now, screening can be done with ultra-large virtual libraries and chemical spaces of drug-like compounds, which can be readily made on-demand, rapidly growing beyond billions of compounds19, and even larger generative spaces with theoretically predicted synthesizability (Box 1). The third factor involves emerging computational approaches that strive to take full advantage of the abundance of 3D structures and ligand data, supported by the broad availability of cloud and graphics processing unit (GPU) computing resources to support these methods at scale. This includes structure-based virtual screening of ultra-large libraries20,21,22, using accelerated23,24,25 and modular26 screening approaches, as well as recent growth of data-driven machine learning (ML) and DL methods for predicting ADMET and PK properties and activities27.
The UC DDC Combines the Drug Discovery Potential of Eight Extraordinary UC Campuses with Six Medical Centers
Nevertheless, most of the lead compounds undergo structural modification to meet their certain biological properties, so-called lead optimization. However, the investigated lead compound has to satisfy five characteristics to act as a bioactive drug molecule. They are potency, duration, safety, availability, and pharmaceutical acceptability, where potency involves the capability of any molecule to exhibit desirable pharmaceutical properties in smaller quantities. Bioavailability marks the transportation of drug compounds with multiple barriers to reach the target site is called bioavailability.
Molecular docking has been greatly facilitated by dramatic growth in computer power and the increasing availability of small molecule and protein databases. Recent advancements in computer methods and access to 3D structural information of biological targets are set to increase the effectiveness of this technique and facilitate its large-scale application to studies of molecular interactions involved in ligand-protein binding. Many molecular docking programs have been developed during recent years, such as, AutoDock [40], Dock [41], FlexX [42], Glide [43], Gold [44], Surflex [45], ICM, and LigandFit [46], and been used successfully in many computer based drug discovery projects. Typically, the major goal of molecular docking is to identify ligands that bind most favorably within receptor binding sites and to determine its most energetically favored binding orientations (poses). A binding pose either refers to a conformation of a ligand molecule within the binding site of its target protein which has been confirmed experimentally, or a computationally modelled hypothetical conformation.
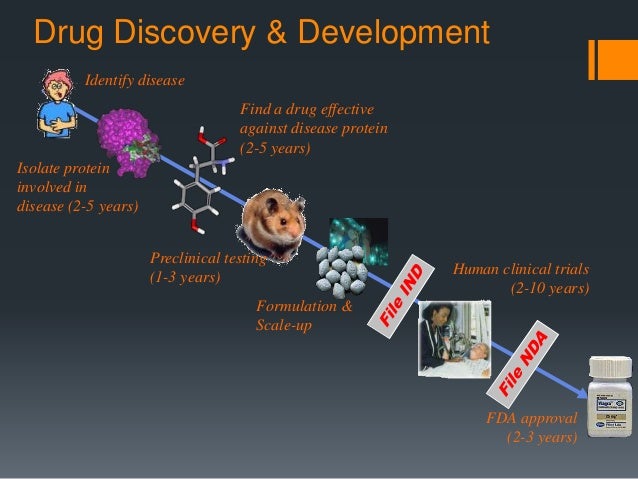
The search algorithm and the scoring function are two important components for determining protein-ligand interactions [47]. The search algorithm is responsible for searching different poses and conformations of a ligand within a given target protein and the scoring function estimates the binding affinities of generated poses, ranks them, and identifies the most favorable receptor/ligand binding modes [47, 48]. An ideal search algorithm should be fast and effective, and the scoring function must be capable of determining the physicochemical properties of molecules and the thermodynamics of interactions. The existing modern drug discovery implies the knowledge of genomics, bioinformatics, computational chemistry, and combinatorial chemistry for virtual screening, HTS, Lenovo ligands. However, the concept of LBDD relies mostly on drug planning, which develops a simple drug molecule, a 3D through trial and error methods that improves the overall biological activity of the compound. Visceral Leishmaniasis (VL) is a serious public health issue, documented in more than ninety countries, where an estimated 500,000 new cases emerge each year.
This technique uses a known active compound as a query compound to find similar compounds and then rank compounds identified in a database. Based on this belief, structurally similar molecules exhibit similar biological activities and physicochemical properties. Numerical descriptors are applied and similarity coefficient is defined to quantify the degree of similarity (similarity/ dissimilarity).
The position and motion of every atom of the system are captured at every point in time, which is quite tough using any experimental technique. MD simulations have been extensively used in the structure-based drug discovery process as this technique helps to unravel many atomistic details such as binding, unbinding, and conformational changes in the receptor at a fine resolution which normally cannot be obtained from experimental studies [59, 60]. Further, using MD simulation it is possible to explore the dynamics of receptor-ligand interactions (association and dissociation) and quantify the thermodynamics, kinetics, and free energy landscape [61]. Following the significant milestone that penicillin represents in human medical history, the battle between humans and bacteria has never settled down and becomes even more vigorous caused by the steady rise of drug resistance.
Readers are highly recommended to refer to the first edition of this chapter (4) for basic CADD concepts and classical protocols to gain a fundamental understanding about CADD methods towards antibiotics development. Pharma companies amass collections of compounds for screening in-house, whereas in-stock collections from vendors (see the figure, part a) allow fast (less than 1 week) delivery, contain unique and advanced chemical scaffolds, are easily searchable and are HTS compatible. However, the high cost of handling physical libraries, their slow linear growth, limited size and novelty constrain their applications.
Cathepsin L is a lysosomal cysteine protease belonging to a family of proteases involved in proteolysis of protein antigens produced by pathogen endocytosis. The protease cleaves the S1 subunit of the coronavirus spike glycoprotein which is required for the virus entry into human host cells, virus, and host cell endosome membrane fusion [128]. These structures solved through experimental techniques or computational homology modeling techniques can be used for structure-based virtual screening for identification of specific inhibitors of the target proteins. Β-lactamase was used as a target in combination therapy against multi-drug resistant Enterobacteriaceae (15). Β-lactamase inhibitors may help to inactivate the β-lactamase enzyme of the pathogen and restore the function of β-lactam antibiotics to overcome the enzyme-mediated resistance. Using the full Site Identification by Ligand Competitive Saturation (SILCS) (16–18) technology developed in our laboratory (Figure 1), we identified β-lactamase CMY-10 inhibitors with our experimental collaborators (19).
Fragment databases from screened ligands for drug discovery (FDSL-DD) - ScienceDirect.com
Fragment databases from screened ligands for drug discovery (FDSL-DD).
Posted: Thu, 28 Dec 2023 03:11:52 GMT [source]
The pipeline involves the prediction of structure, visualization, binding site characterization, molecular docking and virtual screening, visualization of docked complex structure and their stability analysis, ADMET screening, and binding-free energy- MM PBSA will be involved. Computational approaches are useful tools to interpret and guide experiments to expedite the antibiotic drug design process. Structure based drug design (SBDD) and ligand based drug design (LBDD) are the two general types of computer-aided drug design (CADD) approaches in existence. SBDD methods analyze macromolecular target 3-dimensional structural information, typically of proteins or RNA, to identify key sites and interactions that are important for their respective biological functions. Such information can then be utilized to design antibiotic drugs that can compete with essential interactions involving the target and thus interrupt the biological pathways essential for survival of the microorganism(s).
The applicability of CADD for modeling of the drug considers combinatorial chemistry and bioinformatics which address the major issues including cost and time duration. Computational binding site identification methods can be used to exploit novel, druggable sites on new protein targets for potential therapeutic development (113). For antibiotics development, such methods can be employed to search for putative allosteric sites as alternatives to the active or orthosteric sites on bacterial proteins to overcome drug resistance issues (114).
No comments:
Post a Comment